There are few things in life more tedious than assigning boundaries to fundamentally ill-defined concepts. Either terms are used to divide things that cannot be divided (“No, no, that is reddish-purple and clearly not purplish-red!”) or are used to combine groups while ignoring any differences (Republicans? Democrats? just “Washington insiders”). Arguing over the terms is fundamentally unsatisfying: it rarely affects the underlying phenomena.
So when INFORMS (Institute of Operations Research and the Management Sciences), an organization of which I am proud to have been President and equally proud to be Fellow, embarks on its periodic nomenclature debate, ennui overwhelms. Not again! The initial debate between Operations Research and Management Science resulted in two societies (ORSA and TIMS) for forty years before they combined to form INFORMS in 1995. Decision Engineering, Management Engineering, Operations Engineering, Management Decision Making, Information Engineering, and countless other terms have been proposed at times, and some have even made toeholds in the form of academic department names or other usages. None of this has fundamentally changed our field, except perhaps in confusing possible collaborators and scaring off prospective members (“Wow, if they don’t even know who they are then maybe I should check out a more with-it field!”). I decided long ago to just stick with “operations research” and make faces of disgust whenever anyone wanted to engage the issue of the name of the field.
Then, three years ago (only! check the google trends graph) the phrase “business analytics” came along, and it was a miracle! Here was the phrase that really described what we were doing: using past data to predict the future and make better business decisions based on those predictions. That’s us! And, due to books such as “Competing on Analytics”, the wider world actually were interested in us! There were even popular books like “The Numerati” about us. We were finally popular!
Except it wasn’t really about “us” in operations research. We are part of the business analytics story, but we are not the whole story, and I don’t think we are a particularly big part of the story. A tremendous amount of what goes by the name “business analytics” are things like dashboards, business rules, text mining, predictive analytics,OLAP, and lots of other things that many “operations research” people don’t see as part of the field. IBM’s Watson is a great analytics story, but it is not fundamentally an operations research story. People in these areas of business analytics don’t see themselves as doing operations research. Many of them don’t even identify with business analytics but rather with data mining, business intelligence, or other labels. All of this involves “using past data to help predict the future to make better decisions” but “operations research” doesn’t own that aspect of the world. There are lots of people out there who see this as their mandate but haven’t even heard of operations research, and really don’t care about that field.
This is not surprising for those with an INFORMS-centric point of view. INFORMS does not (and near as I can tell, ever has) represent even all of “operations research”. According to the Bureau of Labor Statistics, there are more than 65,000 people with the job “operations research analyst”. INFORMS membership of a bit more than 10,000 is a small fraction of all those involved in operations research. INFORMS is not all of operations research: it certainly is a small amount of business analytics. How can INFORMS “own” business analytics when it doesn’t even own operations research?
Recognizing this divide does not mean erecting a wall between the areas (see the first paragraph on the mendacity of labels). I think the “business analytics” world has a tremendous amount to learn from the “operations research” world and vice versa. Here are few things the two groups should know (and are clearly known by some on both sides, though not to an ideal extent); I welcome your additions to these lists:
What Business Analytics People should Learn from Operations Research
- Getting data and “understanding” it is not enough.
- Predicting the future does not imply making better decisions.
- Lots of decisions are interlinked in complicated ways. Simple rules are often not enough to reconcile those linkages.
- Handling risk is more than knowing about the risk or even modeling the risk. See “stochastic optimization”.
- Organizations have been competing on and changed by analytics for a long, long time now. See the Edelman competition to start.
- Operations research is not exactly an obscure field. Check the google trends of “operations research” versus “business analytics” (with OR in blue and BA in red).
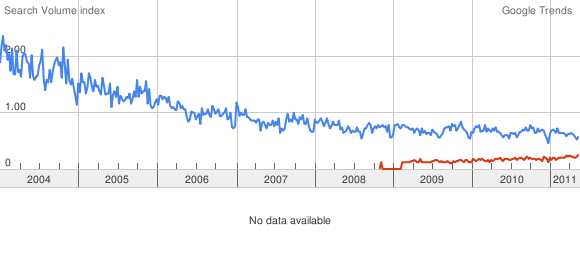
What Operations Research People should Learn from Business Analytics
- It is not just the volume of data that is important: it is the velocity. There is new data every day/hour/minute/second, making the traditional OR approach of “get data, model, implement” hopelessly old-fashioned. Adapting in a sophisticated way to changing data is part of the implementation.
- Not everything is complicated. Sometimes just getting great data and doing predictions followed by a simple decision model is enough to make better decisions. Not everything requires an integer program, let alone a stochastic mixed integer nonlinear optimization.
- Models of data can involve more than means and variances, and even more than regression.
- One project that really changes a company is worth a dozen papers (or perhaps 100) in the professional literature.
- It is worthwhile for people to write about what is done in a way that real people can read it.
I believe strongly in both operations research and business analytics. I have spent my career advancing “operations research” and have never shied from that name. And I just led an effort to start an MBA-level track in business analytics track at the Tepper School. This track includes operations research courses, but includes much more, including courses in data mining, probabilistic marketing models, information systems, and much more.
The lines between operations research and business analytics are undoubtedly blurred and further blurring is an admirable goal. The more the two worlds understand each other, the more we can learn from each other. INFORMS plays a tremendously important role in helping to blur the boundaries both by sharing the successes of the “operations research world” with the “business analytics” world, and by providing a conduit for information going the other way. And this, more than “owning” business analytics, is what INFORMS and its members should be doing.
Ironically, this is part of the INFORMS Blog Challenge.